Gurucul User and Entity Behavior Analytics
Recognized in the Category:
Photo Gallery
![]() |
![]() |
Gurucul User and Entity Behavior Analytics
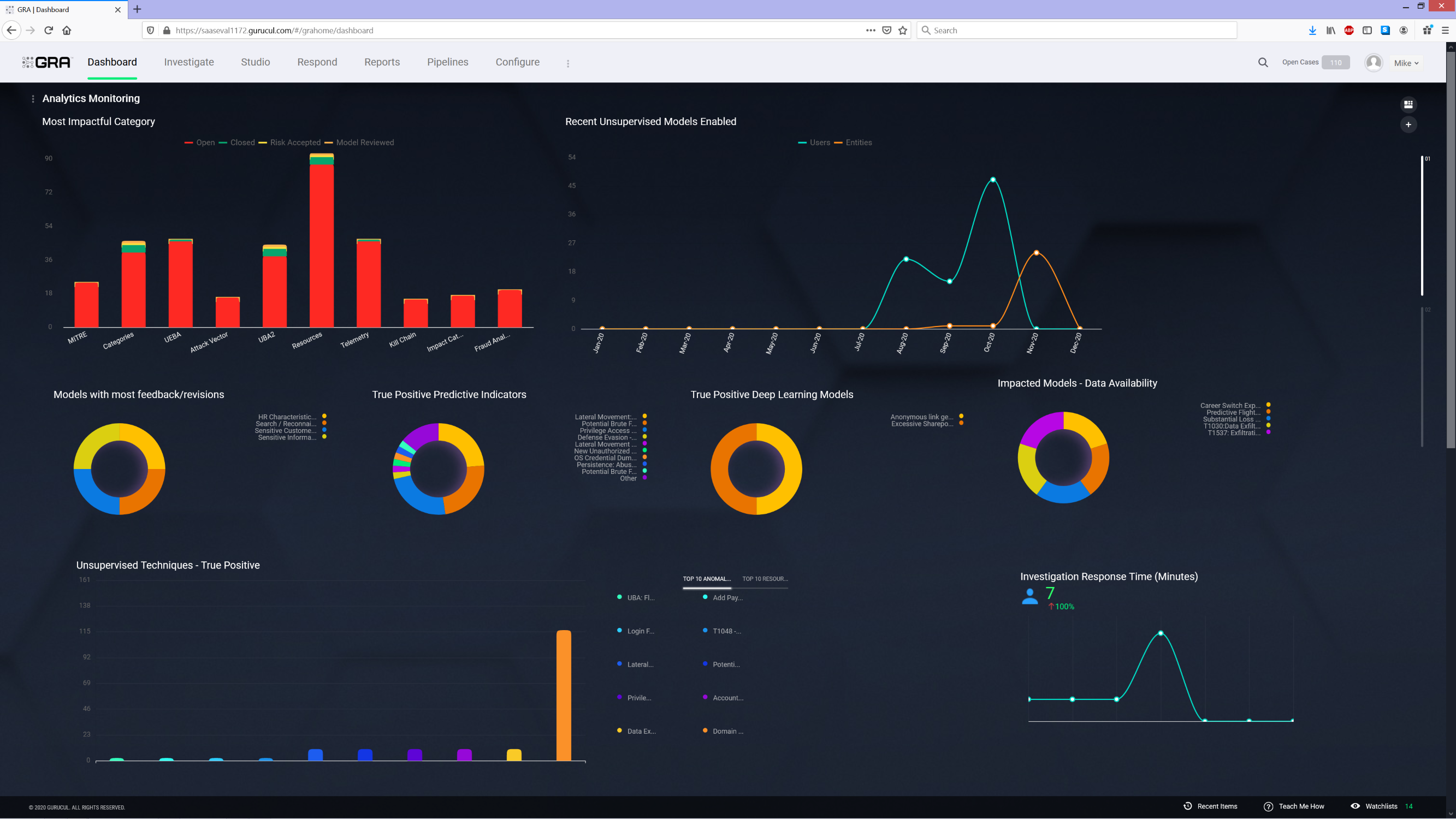
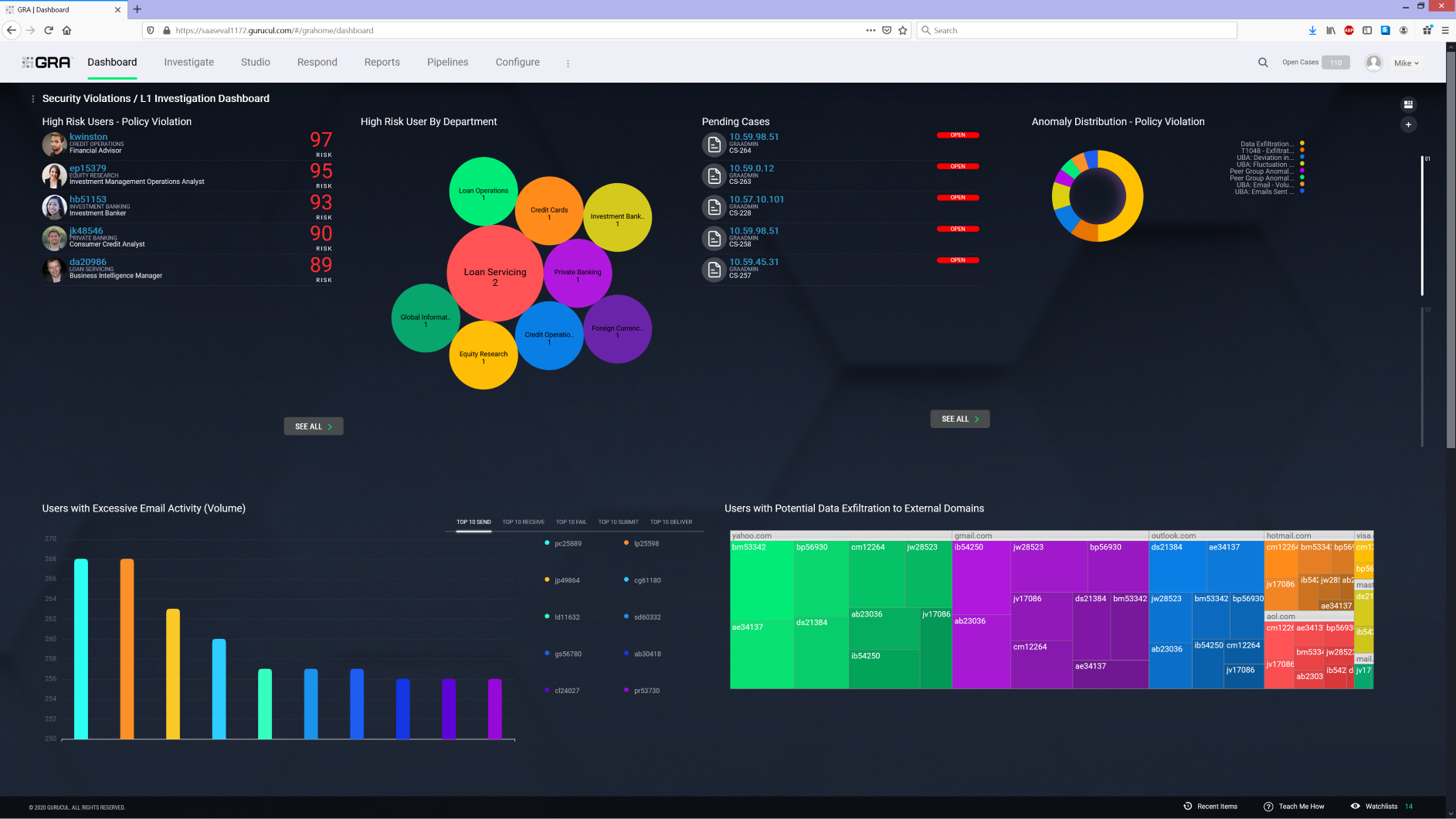
Additional Info
Company | Gurucul |
Company size | 100 - 499 employees |
Website | https://gurucul.com/ |
NOMINATION HIGHLIGHTS
Gurucul was the first to innovate the User and Entity Behavior Analytics (UEBA) category and extend behavior analytics from on-premises to the cloud. Gurucul UEBA goes beyond policies that identify only known threats. By applying big data-based analytics, artificial intelligence and machine learning to user and entity behaviors, Gurucul UEBA identifies unknown threats in real-time that would otherwise appear as “normal” activity to rules-based engines.
Gurucul UEBA ingests and analyzes massive volumes of data generated by user activity from virtually any data source, including the network, IT systems, cloud platforms, applications, IoT, social media, etc. It applies advanced AI and algorithms from the industry’s largest machine learning library (2000+) to hundreds of thousands of discrete events from multiple datasets to identify relationships that can span time, place, and actions. Gurucul UEBA links and analyzes these disparate, obscure, and highly complex behavioral relationships to derive meaning and assess risk for early warning detection, prediction and prevention. Gurucul’s security analytics are aligned with the MITRE ATT&CK framework for advanced threat detection. Gurucul UEBA saves security analyst time and stops damage before it can occur.
Gurucul UEBA solves the challenges organizations face with legacy SIEM, where context for alerts is siloed and there is no linkage between user identities, their accounts, access, and activities, and behavior patterns in applications over time. Gurucul UEBA finds unknown unknowns with no manual threat hunting and no configuration, no writing queries or complex SIEM correlation rules for threats that then requires additional analysis across multiple data sources or applications.
Gurucul UEBA protects against a wide range of threats, including malicious insiders, fraud, account compromise, ransomware, and APT/stealth attacks that lay dormant between stages of a cyberattack. Gurucul even predicts the departure of disgruntled employees, while preventing them from exfiltrating data, committing fraud or sabotaging systems.
How we are different
- Open Analytics: Gurucul STUDIO lets customers adapt Gurucul’s machine learning models or define custom models for their unique requirements, without the need for coding or advanced data science knowledge. Customers can define any information that is stored in Gurucul’s Security Data Lake common information schema as an entity. For example, a sensitive document can be defined as an entity and integrated with Gurucul's Risk based approach to detect any misuse or unauthorized access to the document. There are no restrictions, which gives greater flexibility to customers to define their own entity based risk profiles and monitoring beyond devices, servers, and machines.
- Multistage Analytics: The industry’s first rollout of model chaining to automatically link and visualize the sequence of threats in one screen without manual process or lookups. This technique identifies and creates a dynamic threat chain of anomalies stitching together different stages of attack, with all the necessary metadata and risk associated at each step. It also provides the additional information such as playbook recommendations, total entities affected, log sources involved, users impacted, dwell time, etc. As a part of the workflow, it also allows automated mitigation using built-in playbooks which can be triggered manually. Bidirectional integration with external systems captures the mitigation status and audit information providing a centralized dashboard to track and report the current state of incident response to SOC Manager and executives.
- AI Powered Threat Hunting: Gurucul provides a natural language and model driven threat hunting experience to minimize analyst time writing complex queries. It provides a simple UI driven “point and shoot” experience for investigation teams to build complex queries with inclusions, exclusions, functions, and operators as well as allowing users to select the visualization graphs and charts. It uses AI techniques to identify any additional users/entities impacted which are similar to the
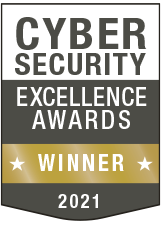
Vote by Sharing
- Like
- Digg
- Tumblr
- VKontakte
- Buffer
- Love This
- Odnoklassniki
- Meneame
- Blogger
- Amazon
- Yahoo Mail
- Gmail
- AOL
- Newsvine
- HackerNews
- Evernote
- MySpace
- Mail.ru
- Viadeo
- Line
- Comments
- SMS
- Viber
- Telegram
- Subscribe
- Facebook Messenger
- Kakao
- LiveJournal
- Yammer
- Edgar
- Fintel
- Mix
- Instapaper
- Copy Link
Each completed social share counts as a vote for this award nomination.