ManageEngine Log360
Photo Gallery
![]() |
ManageEngine Log360
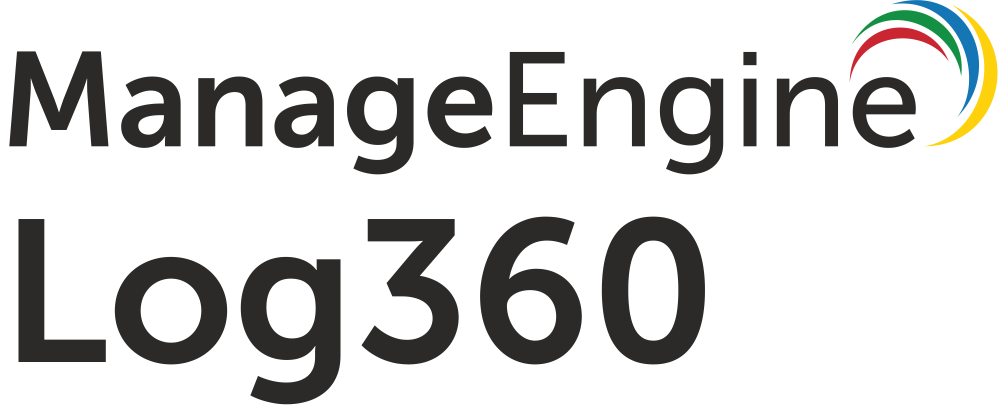
Additional Info
Company | ManageEngine |
Website | https://www.manageengine.com/ |
Company size (employees) | 10,000 or more |
Headquarters Region | North America |
Type of solution | Software |
Overview
Log360 UEBA is powered by machine learning, and can detect anomalies in the behavior of users and entities in a network. There are three types of anomalies that it looks for:
1. Time anomaly: This occurs when a user performs an activity, or an entity has an activity on it, at a time that falls outside an expected range.
2. Count anomaly: This occurs when the number of activities performed by a user (or performed on a host) exceeds an expected threshold.
3. Pattern anomaly: Occurs when an unexpected sequence of events happens together.
Based on the extent of the anomalies, an overall risk score is calculated for every user and host. For users, this overall risk score is further divided into four sub-risk scores – the risk that a user poses for insider threat, data exfiltration, logon anomalies and compromised accounts is displayed. A user’s timeline of anomalous activity can also be easily viewed to understand what took place.
Seasonality factors and dynamic peer group analysis are used for better risk scoring in Log360. While seasonality enables you to disregard expected seasonal behavior deviations while calculating risk, peer group analysis enables you to get the overall context of how a particular user’s behavior compares to other members within their peer group. You can also customize risk scoring by defining the list of anomalous activities that need to be considered, the weightage or importance that needs to be given to each anomalous activity, and the time decay factor or the time it takes for a risk score to gradually fall back to zero.
Log360 enables you to set up alerts based on:
1) Discrete anomalous occurrences within the network
2) Risk thresholds defined by your organization’s risk appetite
How we are different
1. Ability to build custom anomaly models: Log360 offers built-in anomaly models that learn the baseline of expected activity for every user and host in the network. For example, to discover time anomalies, Log360's built-in anomaly model looks for what is normal at 15-minute intervals,o discover count anomalies, it aggregates the number of user and host activities every hour. In addition to this, Log360 allows you to train your own anomaly model using different time intervals and aggregation periods. This capability is called custom anomaly modeling. It can enable you to cater to the specific security situation of your company in a better way.
2. Consideration of seasonality for better risk scoring: Log360 scores risks accurately by considering seasonality factors. Log360 will learn about any activity that happens in a network at regular intervals, whether hourly, daily, weekly or monthly. If an activity that seems normal at first glance is in fact happening at an unusual time, Log360 recognizes it as an anomaly.
3. Peer group analysis for better risk scoring: Log360 identifies users or hosts with similar characteristics or behavioral patterns and classifies them as one peer group. You can build better security by comparing the observed behavior of a user or host to that of the relevant peer group. The user or host risk score can be impacted positively or negatively depending on the peer group. Log 360 uses behavior-related information collected over time to build peer groups. If a user exhibits a behavior for the first time, it checks whether a peer group that showcases such a behavior already exists. If it does, the user is classed into that peer group. To calculate the risk score, it then analyzes variables such as the number of members in the peer group and the frequency of occurrence of the action.